Training Data for Self-Driving Cars: The Backbone of Autonomous Technology
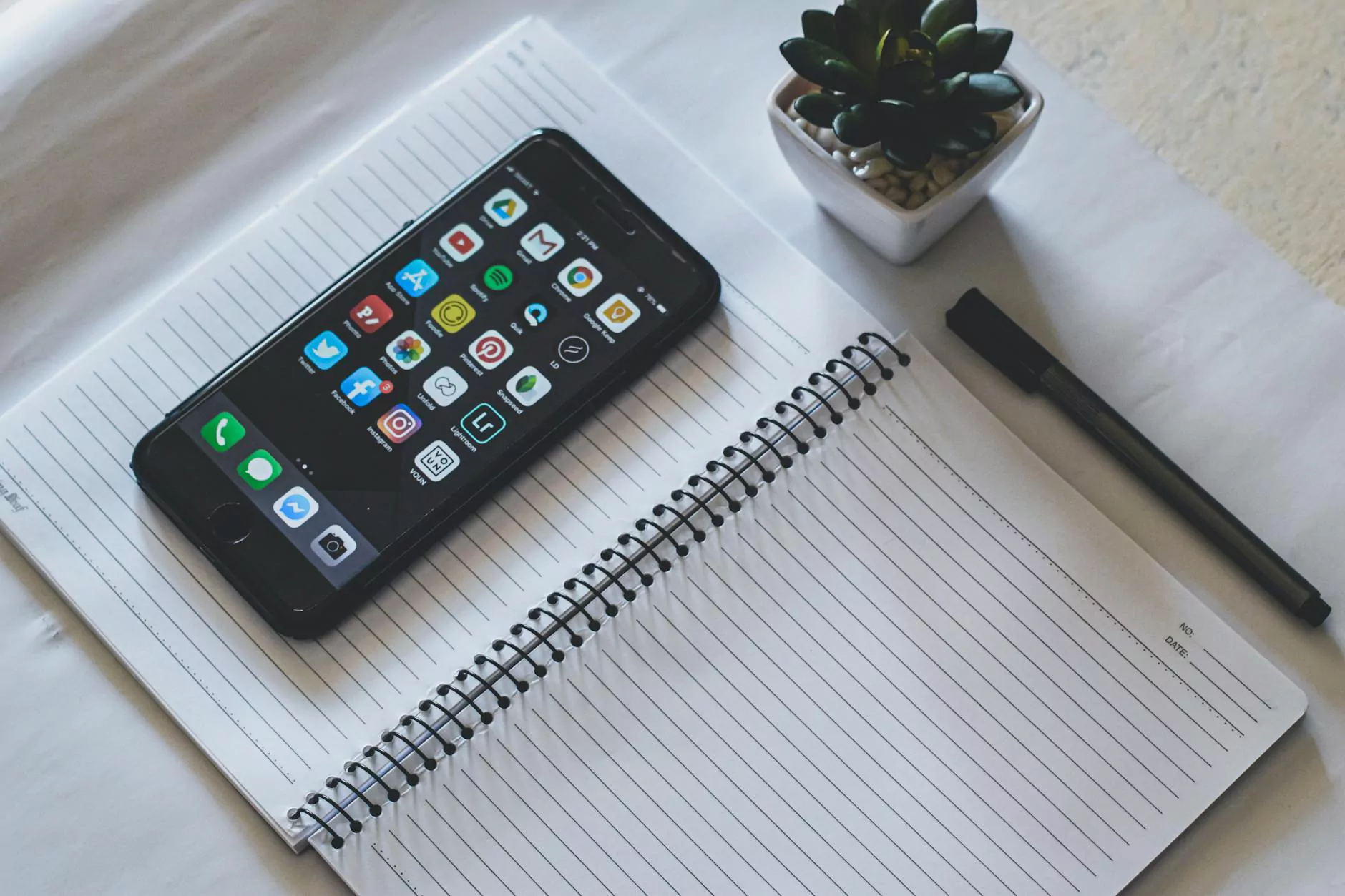
In the rapidly evolving world of automotive technology, self-driving cars represent one of the most significant advancements in recent history. Central to this innovation is the concept of training data for self-driving cars. This article will delve into the importance of high-quality training data, its role in software development, and how companies like keymakr.com are leading the way in creating robust datasets that empower autonomous vehicles.
The Importance of Training Data
Training data is the foundation upon which machine learning models are built. In the context of self-driving cars, this data is crucial for teaching algorithms how to understand and interpret the world around them. Whether it's identifying road signs, detecting pedestrians, or predicting the behavior of other vehicles, rich datasets are essential for developing reliable and safe autonomous driving systems.
1. Types of Training Data
There are several types of training data that contribute to the development of self-driving cars:
- Image and Video Data: Essential for recognizing objects, landmarks, and road conditions.
- Lidar and Radar Data: Provides depth perception and helps in mapping the surroundings.
- Text Data: Used for understanding navigational instructions and user commands.
- Sensor Data: Captures information from various sensors within the vehicle, which enhance decision-making capabilities.
How Training Data is Collected
Collecting quality training data for self-driving cars involves a multifaceted approach that includes both real-world driving experiences and simulated environments. Here’s how these methods work:
2. Real-World Data Collection
To train self-driving algorithms effectively, companies utilize a combination of on-road testing and sensor data logs. During these tests, vehicles are equipped with multiple sensors to gather data in various conditions:
- Urban Environments: Capturing data in busy city streets with pedestrians, cyclists, and various traffic signs.
- Rural Areas: Understanding how to handle less congested roads and wildlife.
- Adverse Weather Conditions: Testing in rain, snow, and fog to ensure reliability in all conditions.
3. Simulation Environments
Simulators play a critical role in augmenting real-world data collection. They allow developers to create scenarios that are difficult or dangerous to recreate in the real world:
- Crash Scenarios: Testing the limits of the autonomous systems without risking lives.
- Rare Events: Simulating unusual driving conditions that seldom occur but pose significant safety risks.
The Role of Software Development in Training Data
Software development is at the heart of processing the vast amount of training data collected. Machine learning engineers and data scientists work together to refine algorithms that learn from this data. Here’s how the process typically works:
4. Data Annotation
Before data can be used for training, it must be annotated or labeled accurately. Annotation involves tagging images and videos with metadata to provide context. For instance, identifying vehicles, traffic signs, and pedestrians is essential for the algorithms to learn correctly.
5. Model Training
After data is collected and annotated, machine learning models undergo a training phase. In this phase, algorithms learn to recognize patterns and make predictions based on the input data. This is often achieved through methods such as:
- Neural Networks: Particularly deep learning networks that can process vast amounts of information.
- Reinforcement Learning: Where an agent learns to make decisions through trial and error.
Challenges in Training Data for Self-Driving Cars
Despite advancements, there are substantial challenges in obtaining and utilizing training data:
6. Ensuring Data Quality
Not all collected data is useful. Ensuring that the data is accurate, comprehensive, and free from bias is crucial. Inaccurate data could lead to poor decision-making by autonomous systems.
7. Data Privacy and Security
With the collection of data comes the responsibility to ensure that user privacy is maintained. Autonomous vehicles often gather sensitive information, and companies must adhere to strict regulations to protect user data.
Innovations in Training Data Techniques
Companies at the forefront, such as keymakr.com, are pioneering new methodologies in training data techniques to enhance the capabilities of self-driving cars:
8. Crowd-Sourcing Data Annotation
Leveraging the power of crowdsourcing allows companies to scale their data annotation efforts quickly. By distributing the task of labeling data across a large group of individuals, organizations can significantly reduce costs and increase the speed of data preparation.
9. Synthetic Data Generation
Synthetic data, created through simulations or data augmentation techniques, can complement real-world data. This approach helps to bridge gaps in datasets, particularly in rare scenarios that are difficult to capture. Furthermore, it allows for the creation of diverse training environments that can enhance the robustness of the models.
The Future of Training Data in Autonomous Driving
As technology continues to advance, the methods of collecting and utilizing training data will also evolve. Future trends may include:
10. Improved Data Integration
Integrating data from various sources, such as traffic management systems and other vehicles (V2V communication), can enrich the datasets and provide a more comprehensive understanding of driving environments.
11. Increased Focus on Ethics in AI
As algorithms become more sophisticated, there will be a greater emphasis on ethical considerations in AI development, including the impact of biased training data and the need for transparency in how decisions are made.
Conclusion
In conclusion, training data for self-driving cars is not merely a technical requirement; it is the lifeblood of the entire autonomous driving revolution. The quality and diversity of training data will ultimately determine the success of self-driving technology in delivering safe, reliable, and efficient transportation solutions. Organizations like keymakr.com are crucial players in this domain, ensuring that the software development efforts around autonomous vehicles are grounded in robust, quality data.
As we advance towards a future dominated by autonomous vehicles, the ongoing innovation in data collection, processing, and utilization will play a pivotal role in shaping the landscape of mobility.
training data for self driving cars